SUMMARY
Combining Computer-Aided Engineering (CAE) with real-world measured data unlocks new possibilities in engineering and manufacturing. CAE provides powerful simulations to predict system behavior, while measured data enhances accuracy by validating and refining these models. This integration improves simulation reliability, enables predictive maintenance, optimizes processes in real-time, and fosters continuous improvement. Applications span industries like automotive, aerospace, energy, and manufacturing, where it enhances product quality, operational efficiency, and decision-making. Together, CAE and measured data offer a dynamic approach to data-driven engineering, delivering deeper insights and driving innovation in design and production.
INDUSTRY
automotive, aerospace, energy, manufacturing, production
RESOURCES
In the world of engineering and manufacturing, data-driven decision-making is more crucial than ever. Combining Computer-Aided Engineering (CAE) with measured data from real-world processes allows businesses to gain deeper insights, improve accuracy, and optimize performance. In this blog post, we'll explore how and why CAE, when integrated with measured data, offers a powerful approach to achieving more reliable and effective results.
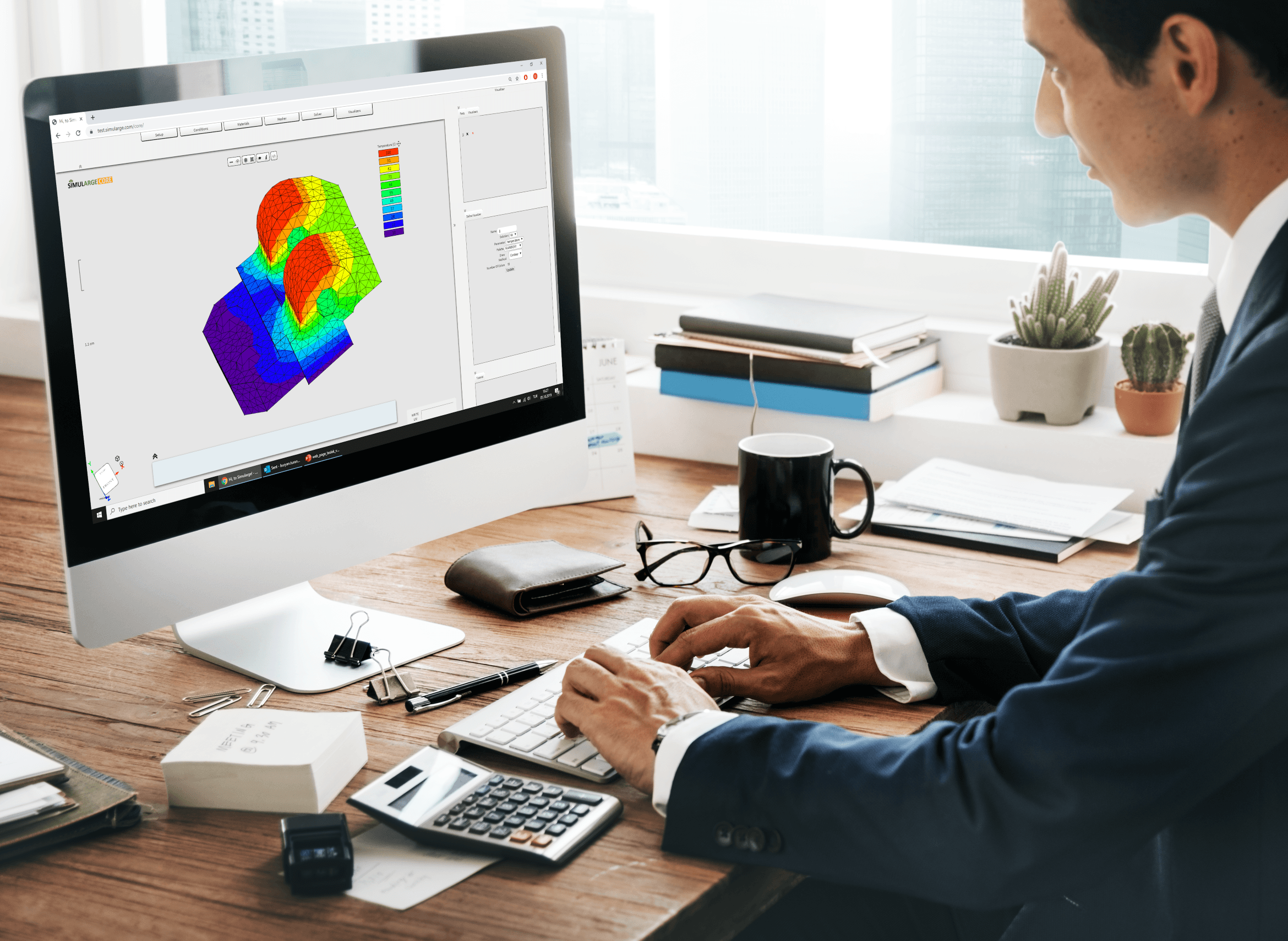
What is CAE and How Does It Work?
Computer-Aided Engineering (CAE) involves using software to simulate and analyze the physical behavior of products or systems under various conditions. Whether it's structural analysis, fluid dynamics, or thermal performance, CAE helps engineers predict outcomes, reduce risks, and make informed design decisions before products are manufactured. It allows engineers to test designs virtually, reducing the need for physical prototypes and iterations.
However, CAE simulations are only as accurate as the models and assumptions used. This is where the combination of CAE with measured data comes into play, closing the gap between virtual simulations and real-world performance.
Why Combine CAE with Measured Data?
Increased Simulation Accuracy CAE relies on assumptions, boundary conditions, and material models to simulate performance. While these inputs are valuable, measured data from actual processes (such as sensor data from manufacturing equipment) can provide real-time insights that help refine these assumptions. This leads to simulations that better reflect reality and enhance the accuracy of predictions.
Validation and Calibration of Models One of the main challenges with CAE is ensuring that the simulation models are accurate. Measured data from physical tests allows engineers to validate and calibrate their models. For example, data from strain gauges, temperature sensors, or flow meters can be fed into the CAE software to compare simulation results with actual performance, identifying discrepancies and making necessary adjustments.
Enhanced Predictive Maintenance By combining CAE with real-time sensor data from production equipment, manufacturers can predict potential failures before they happen. For example, if a CAE model simulates how a machine component should behave under certain stress conditions, measured data can show deviations from this expected performance, indicating wear or potential failure. This approach enables predictive maintenance, reducing downtime and costs associated with unexpected breakdowns.
Optimized Process Control In production environments, integrating CAE with measured data helps optimize process parameters. For instance, in metal forging or plastic injection molding, the behavior of materials under specific pressures and temperatures can be modeled using CAE. However, by incorporating measured data such as temperature or pressure readings from actual machines, manufacturers can fine-tune their processes in real time, achieving better product quality and higher efficiency.
Continuous Improvement and Adaptation Combining CAE with measured data allows for a continuous feedback loop between design, simulation, and actual production. As measured data is collected, it can be fed back into the CAE models to improve future simulations. This iterative process enables ongoing optimization of both product design and manufacturing processes.
Better Decision-Making with Real-Time Data In industries like automotive, aerospace, and energy, engineers often face complex challenges where decisions need to be made quickly. The combination of CAE simulations with real-time measured data provides an actionable insight into current conditions, allowing decision-makers to respond faster and with greater confidence.
How CAE and Measured Data Work Together
Data Collection The first step is to collect measured data from sensors and equipment in the production environment. This can include temperature, pressure, strain, vibration, and more, depending on the application.
Data Integration The measured data is then integrated into the CAE environment. Modern CAE tools allow for direct input of sensor data, enabling simulations to be run based on actual conditions. This integration helps create models that more accurately reflect real-world behavior.
Simulation and Analysis With the measured data in place, engineers can run simulations to predict how systems will behave under different scenarios. For example, in a thermal simulation, measured temperature data can be used to refine heat transfer models, improving the simulation’s accuracy.
Validation and Adjustment The next step is to compare the simulation results with the measured data to identify any differences. If the simulation and the real-world data do not match, the model can be adjusted, and further simulations can be run to achieve better alignment.
Iterative Optimization Once the simulation has been validated against measured data, it can be used to optimize the system or process. Engineers can test different design variations or process conditions in the virtual environment, using the validated model to find the optimal solution.
Real-World Applications of CAE and Measured Data Integration
Automotive Industry:
In the automotive sector, CAE is used extensively for vehicle design and performance testing. By combining CAE with real-world driving data, manufacturers can improve fuel efficiency, optimize aerodynamics, and enhance crashworthiness. For example, sensor data from crash tests is used to fine-tune safety models, leading to safer vehicles.
Aerospace Industry:
In aerospace engineering, combining CAE with measured data helps ensure the structural integrity of components and systems. Real-time data from aircraft sensors is fed into CAE models to monitor stress and fatigue during flight, helping to predict potential failures and optimize maintenance schedules.
Energy Sector:
In power plants and renewable energy installations, measured data such as temperature, pressure, and flow rates are used to optimize the efficiency of turbines, boilers, and other critical systems. CAE simulations help predict the impact of different operating conditions, while the integration of measured data ensures that the models are accurate and reliable.
Manufacturing and Production:
In manufacturing, combining CAE with data from the production floor allows companies to optimize processes such as metal forming, plastic molding, and additive manufacturing. Measured data ensures that simulations reflect actual material behavior, resulting in improved product quality and reduced waste.
Conclusion: The Future of Data-Driven Engineering
The integration of CAE with measured data offers a powerful synergy that enhances accuracy, optimizes processes, and drives innovation. In an increasingly data-driven world, combining virtual simulations with real-world data provides a level of insight that was previously unattainable. By leveraging this combination, engineers can design better products, streamline production processes, and ultimately achieve greater efficiency and reliability.
Are you ready to unlock the full potential of CAE and measured data integration? Contact us to learn more about how we can help you leverage the power of data-driven engineering solutions.