SUMMARY
In the AI and machine learning era, synthetic data generation is revolutionizing dataset creation by providing cost-effective, privacy-compliant, and diverse data. Unlike real-world data, synthetic data is artificially produced through simulations and algorithms, offering controlled scenarios and scalability. Computer-Aided Engineering (CAE) plays a pivotal role in generating high-quality synthetic data by simulating physical processes, creating diverse scenarios, and ensuring accuracy. Applications range from manufacturing optimization to thermal management in electronics, HVAC systems, and energy generation. Despite its benefits, challenges like ensuring real-world fidelity and managing computational demands remain. Synthetic data generation with CAE is a game-changer, driving innovation and enhancing AI model performance across industries.
INDUSTRY
manufacturing, electronics, automotive, aerospace, energy
RESOURCES
In today’s data-driven landscape, the demand for high-quality datasets is more critical than ever, particularly in the realms of artificial intelligence (AI) and machine learning (ML). Traditional data collection methods can be time-consuming, costly, and sometimes insufficient for training robust AI models. Enter synthetic data generation—a powerful approach that leverages Computer-Aided Engineering (CAE) techniques to create realistic and diverse datasets for AI applications. In this blog post, we will explore the concept of synthetic data generation, its benefits, and how CAE plays a pivotal role in this process.
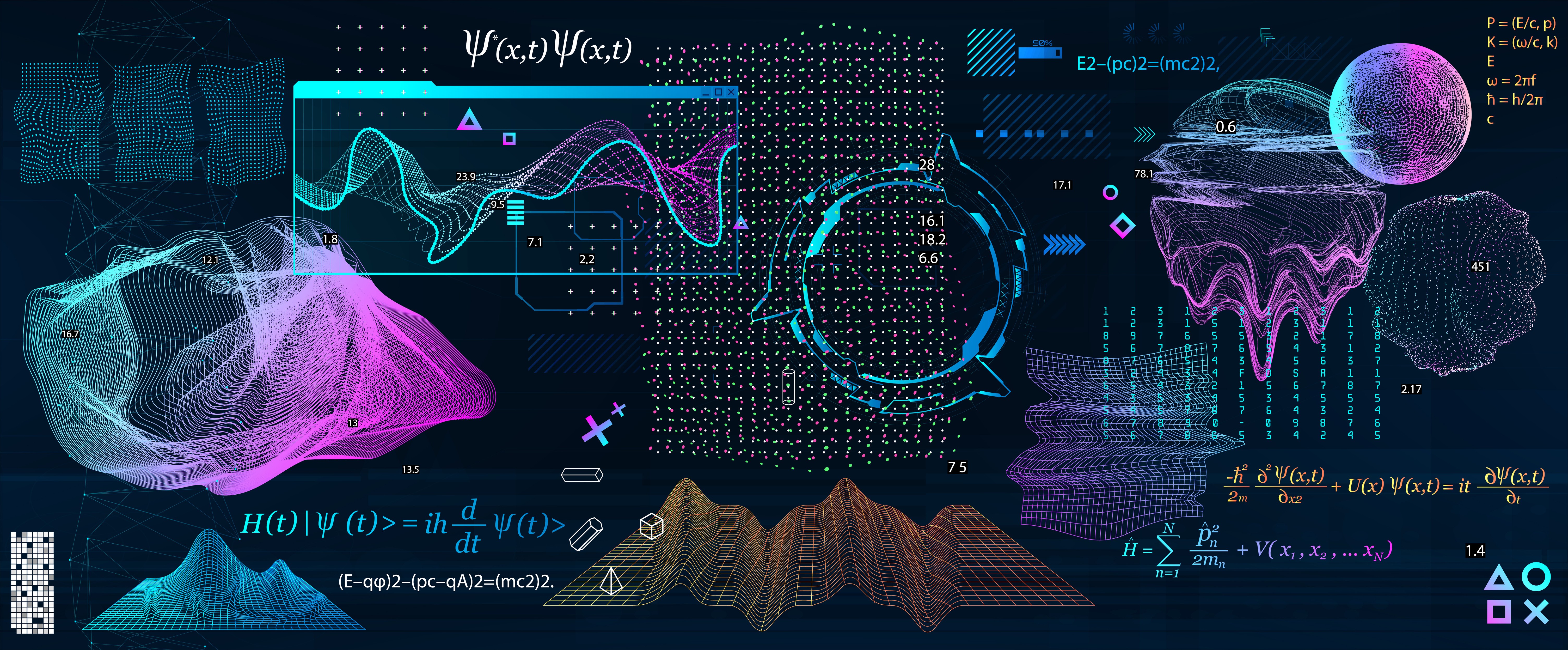
What is Synthetic Data?
Synthetic data refers to data that is artificially generated rather than obtained from real-world events. It mimics the statistical properties and characteristics of real datasets while being devoid of sensitive information or privacy concerns. This data can be created using various techniques, including simulations, algorithms, and statistical models.
Why Use Synthetic Data?
Cost-Effectiveness: Generating synthetic data can be significantly cheaper than collecting real-world data, especially in scenarios where data is scarce or expensive to obtain.
Data Privacy: Synthetic data eliminates privacy concerns associated with real datasets. Organizations can use this data without fear of exposing sensitive information, making it particularly valuable in fields like healthcare and finance.
Augmented Datasets: Synthetic data can be used to augment existing datasets, providing additional variations and increasing the diversity of training data. This can improve the robustness and generalization of AI models.
Controlled Conditions: When generating synthetic data, researchers can control variables and create specific scenarios that may be difficult or impossible to capture in the real world. This capability allows for comprehensive testing and training of AI systems.
The Role of CAE in Synthetic Data Generation
Computer-Aided Engineering (CAE) provides powerful tools and methodologies for simulating real-world physical phenomena. These simulations can be instrumental in generating synthetic data for AI applications. Here’s how CAE contributes to synthetic data generation:
Simulation of Physical Processes: CAE allows engineers to model and simulate complex physical processes, such as fluid dynamics, heat transfer, and structural behavior. By running simulations under varying conditions, CAE can produce vast amounts of data that reflect real-world behaviors.
Creation of Diverse Scenarios: Using CAE, engineers can create a wide range of scenarios that account for different variables, including environmental conditions, material properties, and operational parameters. This diversity enhances the quality of the synthetic data generated.
Validation and Accuracy: CAE provides a high level of accuracy in simulations, ensuring that the synthetic data closely resembles real-world data. This accuracy is crucial for training AI models, as it increases the likelihood that the models will perform well in practical applications.
Iterative Learning: As AI models are trained on synthetic data generated through CAE, they can provide feedback that can be used to refine the simulations further. This iterative process enhances both the AI models and the synthetic data generation techniques.
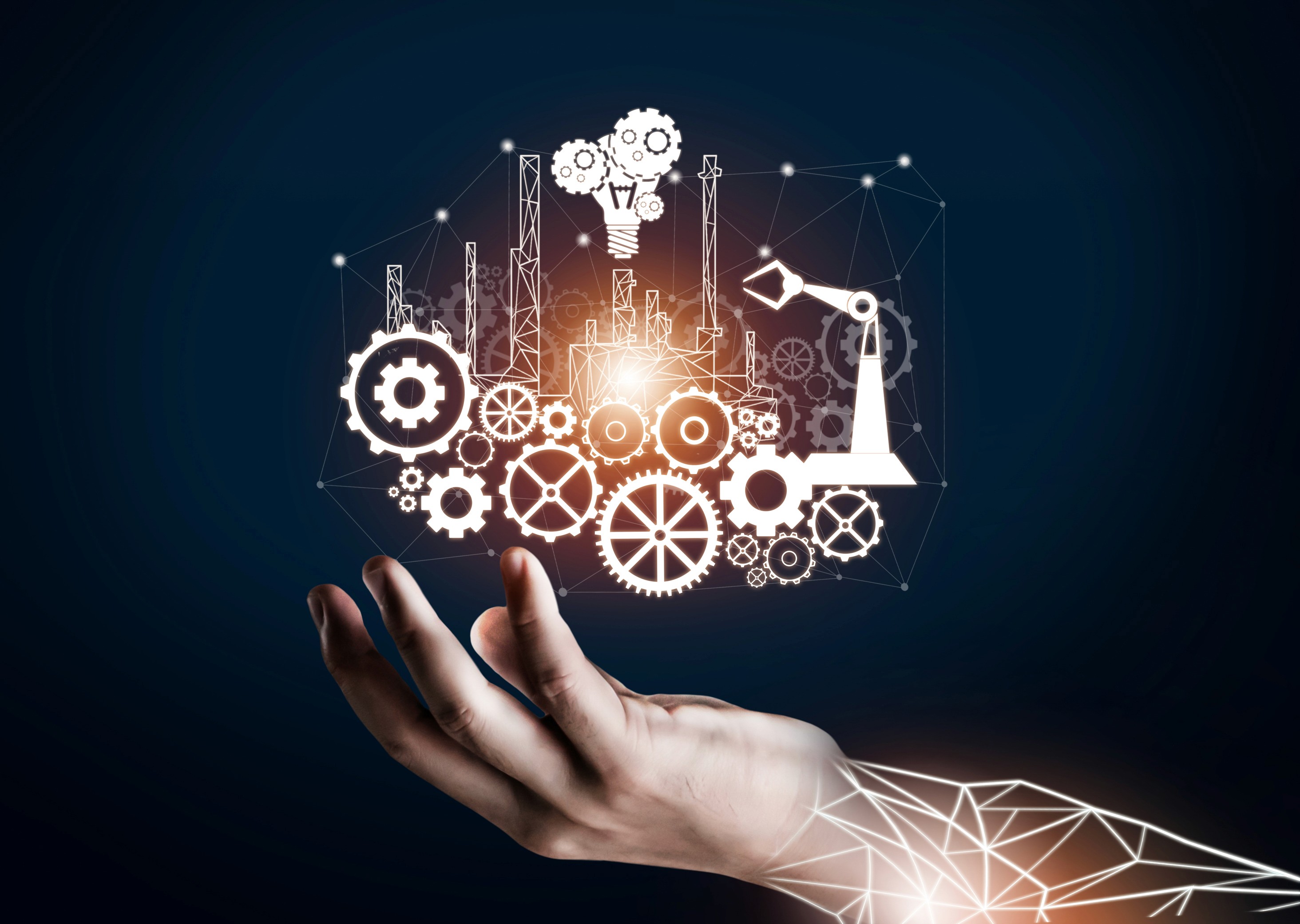
Applications of Synthetic Data in AI with CAE
Manufacturing Process Optimization
Synthetic data generated from CAE simulations in manufacturing can help optimize production processes, such as thermal management in welding or molding, fluid dynamics in extrusion, or structural analysis in assembly lines. By using CAE tools to simulate different process conditions, engineers can create vast datasets for AI models to predict defects, improve material usage, and fine-tune parameters like temperature, pressure, or cooling rates.
Heat Transfer in Electronics Cooling
In industries like consumer electronics and automotive, synthetic data can be generated by simulating heat transfer within devices or systems. For instance, thermal management simulations of electronic components using CAE can produce datasets that allow AI models to optimize cooling solutions, ensure thermal stability, and predict potential overheating issues. This combination of synthetic data and AI accelerates innovation in designing more efficient cooling systems.
Fluid Dynamics for HVAC and Aerodynamics
CFD simulations are key in the design of systems like HVAC (Heating, Ventilation, and Air Conditioning) or aerodynamic analysis in the aerospace or automotive industries. By generating synthetic data through various airflow, pressure, and heat distribution scenarios, engineers can train AI models to predict performance outcomes. This data helps optimize designs for energy efficiency, airflow control, and even emissions reductions in the case of combustion systems.
Turbomachinery and Fluid Flow in Energy Generation
In the energy sector, synthetic data from CAE simulations is crucial for optimizing turbomachinery performance, such as gas turbines or wind turbines. By simulating fluid flow and heat transfer within these systems, engineers can train AI models to predict efficiency losses, wear patterns, or maintenance needs. This leads to enhanced performance, reduced downtime, and better energy production optimization.
Challenges and Considerations
While synthetic data generation presents numerous advantages, it also comes with challenges. Ensuring that the synthetic data accurately reflects real-world scenarios is crucial; otherwise, the AI models trained on this data may not perform well in practice. Additionally, the computational resources required for high-fidelity simulations can be significant, necessitating investment in technology and infrastructure.
Conclusion
Synthetic data generation using CAE offers a powerful solution for overcoming the limitations of traditional data collection methods in AI applications. By leveraging simulation capabilities, organizations can create diverse, accurate, and privacy-compliant datasets that enhance the training and performance of AI models. As technology continues to evolve, the integration of synthetic data generation with CAE will play an increasingly vital role in driving innovation across various industries.
If you're interested in exploring how synthetic data generation can benefit your AI initiatives, feel free to reach out for more information!